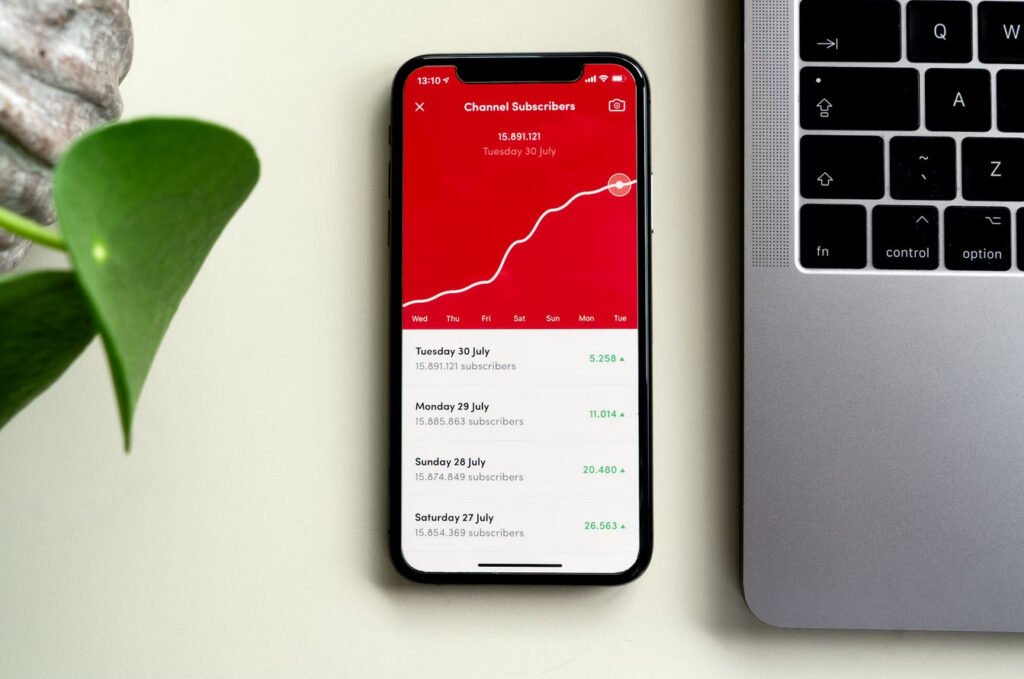
In the ever-evolving landscape of data science, statistics serves as a fundamental pillar, influencing decision-making processes and enabling businesses to extract valuable insights from vast datasets. As companies invest billions in statistics and analytics, the demand for skilled professionals in this field continues to rise.
To help you navigate the intricacies of statistics and prepare for interviews, we’ve compiled a list of key questions and answers that showcase the depth and breadth of this fascinating discipline.
Basic Interview Questions on Statistics
Q: What criteria do we use to determine the statistical importance of an instance?
A: The statistical significance of an insight is determined through hypothesis testing. This involves formulating null and alternate hypotheses, calculating p-values, and comparing them to the chosen alpha level. If the p-value is smaller than alpha, the null hypothesis is rejected, indicating statistical significance.
Q: What are the applications of long-tail distributions?
A: Long-tail distributions, characterized by a diminishing tail in the curve, find applications in the Pareto principle, product sales distribution, and classification and regression problems. They offer insights into the occurrence of rare events.
Q: Define the central limit theorem and its application.
A: The central limit theorem states that, as sample size increases, the distribution of sample means approaches a normal distribution, regardless of the population distribution. It is crucial for hypothesis testing and accurately calculating confidence intervals.
Q: Differentiate between observational and experimental data in statistics.
A: Observational data comes from studies where variables are observed for potential relationships, while experimental data is derived from controlled investigations where specific factors are manipulated to observe changes in outcomes.
Q: What is mean imputation for missing data, and what are its disadvantages?
A: Mean imputation involves replacing null values with the mean of the dataset. Its disadvantages include erasing feature correlations, introducing bias, and reducing model accuracy by increasing variance.
Q: Define an outlier and describe methods for identifying them in a dataset.
A: Outliers are data points significantly different from the rest. Identification methods include the Interquartile range (IQR) and Standard Deviation/Z-score, which highlight data points deviating significantly from the norm.
Q: How is missing data treated in statistics?
A: Several approaches exist for handling missing data, including prediction, individual value assignment, deletion of rows with missing data, mean or median imputation, and using algorithms like random forests for imputation.
Q: What is exploratory data analysis, and how does it differ from other types of data analysis?
A: Exploratory data analysis involves investigating data to identify patterns, anomalies, and confirm assumptions. It differs from other types of data analysis by focusing on initial exploration rather than specific hypothesis testing.
Q: Define selection bias and its implications.
A: Selection bias occurs when non-random selection of data influences model functionality. If proper randomization is not conducted, the sample may not accurately represent the population, leading to biased results.
Q: List the various kinds of statistical selection bias.
A: Types of selection bias include protopathic bias, observer selection, attrition, sampling bias, and time intervals, each influencing data interpretation and analysis.
Q: Define an inlier and its significance in data analysis.
A: An inlier is a data point on the same level as the rest of the dataset. While outliers can reduce model accuracy, the identification and removal of inliers are crucial to ensure continuous model accuracy.
Q: Describe a situation where the median is superior to the mean.
A: The median is preferable when dealing with datasets containing outliers that could skew the data either positively or negatively. The median is less influenced by extreme values and provides a robust measure of central tendency in such situations.
Q: Provide an example of a root cause analysis.
A: Root cause analysis is a problem-solving technique identifying the fundamental cause of a problem. For instance, linking a city’s higher crime rate to increased sales of red-colored shirts does not imply causation. Additional methods like A/B testing or hypothesis testing are needed to assess causality.
Q: What does the term “six sigma” mean?
A: Six Sigma is a quality assurance approach used in statistics to improve processes and functionality. A process is considered six sigma when 99.99966 percent of its outputs are defect-free, indicating high quality and efficiency.
Q: Define DOE in statistics.
A: Design of Experiments (DOE) refers to the task design specifying data and variables’ variation when independent input factors change. It is a crucial aspect of experimental design to understand the impact of variables on outcomes.
Q: Which data types do not have a log-normal or Gaussian distribution?
A: Exponential distributions do not exhibit log-normal or Gaussian characteristics. They are prevalent in scenarios like the duration of a phone call, time until the next earthquake, etc.
Q: Explain the concept of the five-number summary in statistics.
A: The five-number summary comprises the minimum, first quartile (Q1), median, third quartile (Q3), and maximum. It provides a comprehensive view of the dataset’s spread and central tendency.
Q: What is the Pareto principle, and how is it applied in statistics?
A: The Pareto principle, or the 80/20 rule, states that 80% of results come from 20% of causes. In statistics, it is applied to understand that a significant portion of effects may be attributed to a minority of contributing factors.
These interview questions and answers offer a glimpse into the multifaceted world of statistics. As the demand for data-driven insights continues to grow, a solid foundation in statistics is essential for professionals aspiring to make significant contributions to the field of data science.
Whether you’re exploring the intricacies of probability distributions, mastering hypothesis testing, or delving into the world of experimental design, a strong understanding of statistical concepts will undoubtedly open doors to exciting opportunities in the ever-expanding realm of data science.
You may also like:- 80 Most Important Network Fundamentals Questions With Answers
- 100 Most Important SOC Analyst Interview Questions
- Top 40 Cyber Security Questions and Answers
- Top 10 React JS Interview Theory Questions and Answers
- CISSP – Practice Test Questions – 2024 – Set 20 (53 Questions)
- Part 2: Exploring Deeper into CCNA – Wireless (145 Practice Test Questions)
- Part 1: Mastering CCNA – Wireless (145 Practice Test Questions)
- [1z0-1085-20] Oracle Cloud Infrastructure Foundations 2020 Associate MCQ Questions – Part 3
- [1z0-1085-20] Oracle Cloud Infrastructure Foundations 2020 Associate MCQ Questions – Part 2
- [1z0-1085-20] Oracle Cloud Infrastructure Foundations 2020 Associate MCQ Questions – Part 1